The Importance of Data Quality cannot be overlooked in this data-driven era. With vast amounts of data now available, companies, are focused on exploiting data for gaining a competitive advantage over others. We live in the era of Big Data, where the sheer volume and variety of data have far outstripped the capacity of manual analysis. In some cases, even exceeded the capacity of conventional databases. Consequently, the need for more processing power has become evident. However, the increased power of computers counters this challenge. Networking has become ubiquitous, and the development of algorithms that can connect datasets has paved the way for broader and deeper analyses. These advancements have led companies to recognize the significance of Data Science and its unlimited potentialities.
Machine Learning and Artificial Intelligence
Machine Learning and Artificial Intelligence are becoming terms used on a daily basis in our working days. A 2020 Deloitte survey found that 67% of companies are using machine learning. And 97% are using or planning to use it in the next year (Getting Smart About Integrating AI Technology).
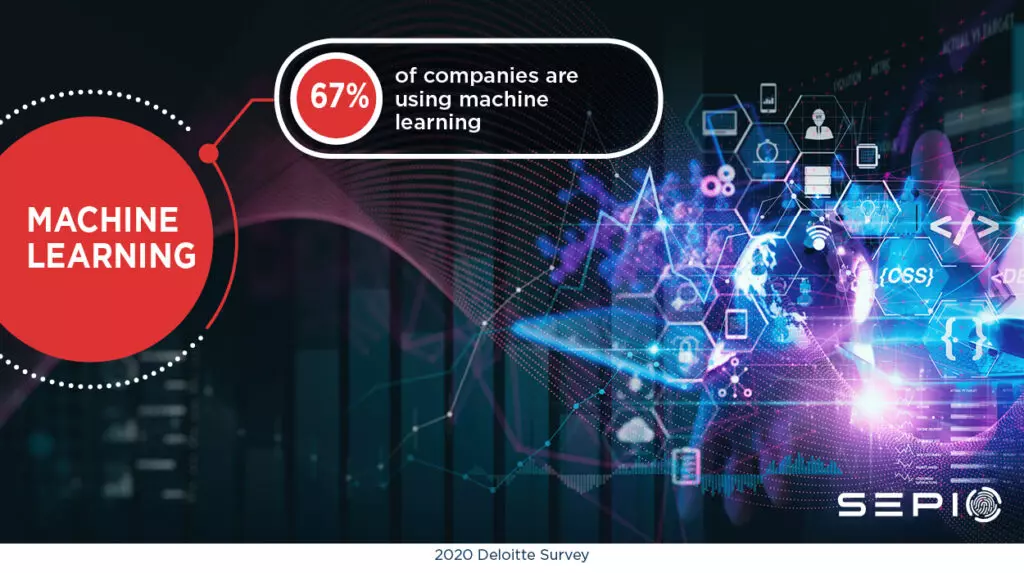
In 1959, Arthur Samuel defined Machine Learning as the subfield of Artificial Intelligence that “gives computers the ability to learn without being explicitly programmed”. Over the last quarter of a century, Machine Learning has become one of the most important parts of the IT revolution impacting our lives.
Although ML dates from the early days of Artificial Intelligence in the late 1950s, it underwent a first resurgence when the concept of data mining began to takeoff approximately 20 years ago. Data mining algorithms look for patterns in information. Machine Learning does the same thing but goes one step further. The program changes its behavior based on what it learns.
Only as Good as the Data They Learn From
Machine Learning starts with data — numbers, photos, text… We collect various types of data from diverse sources and prepare it for use as training data. This data serves as the information on which the machine learning model will be trained. The more diverse the training data is, the better the Machine Learning Algorithm will perform.
But although Machine Learning algorithms can really help leverage a company utilizing its data assets for better results and better products, they will always be as good as the data quality they learn from. If the data they learn from is not diverse enough, is not cleaned or processed, the Machine Learning algorithms can result in overfitting. When a model learns the detail and noise in the training data to the extent that it negatively impacts the performance of the model on new data. In simpler terms, when the data is not diverse and its quality it’s not as high as it could be, Machine Learning models will produce extremely good results in the data they use for training but they will perform poorly on new and unseen data.
Importance of Data Quality and Diversity in Cybersecurity
The importance of data quality and diversity have become an extremely important pillar in any Data Science activity. Especially in hardware cybersecurity where there is no room for small mistakes. At Sepio, we prioritize cleaning and diversifying the data we collect to ensure the maximum performance and success of our Machine Learning models and algorithms.
At Sepio, we understand that the effectiveness of Machine Learning models and algorithms relies heavily on the quality and diversity of the data used for training. We employ rigorous data collection strategies, employing advanced techniques to ensure the data we gather meets the highest standards. This involves thorough validation processes, rigorous data cleansing techniques, and continuous monitoring to maintain data quality over time.
See every known and shadow asset. Prioritize and mitigate risks.
Talk to an expert. It will help you understand how to use Sepio’s patented technology to gain control of your asset risks.